Doesn’t it sound interesting that a computer or device can understand what you speak 🗣? Automatic Speech Recognition (ASR) works like magic🎩, changing your voice into machine-understandable text.
Can you name some devices or applications that use automatic speech recognition? Think about virtual assistants like Siri, Alexa, or Google Assistant, as well as transcription services.
Let’s dig deeper into automatic speech recognition and learn about what automatic speech recognition is, how it works, its benefits, its applications, how its advancements have benefitted us, and the future of automatic speech recognition.
🔑 KEY HIGHLIGHTS
- Automatic speech recognition uses Artificial intelligence or machine learning to communicate and convert human speech into computer understandable format.
- Automatic speech recognition works in a manner that is similar to human interaction by translating sounds into written text.
- ASR makes communication more accessible for people with disabilities, such as those who have difficulty typing or writing.
- The voice assistant is an automatic speech recognition application that takes commands or speech from the user and responds with the voice as per the user’s intent.
What is Automatic Speech Recognition?
Automatic speech recognition is the use of Artificial Intelligence or Machine Learning to communicate with a computer interface using a human voice in the exact same way as a human interaction. This technology processes human speech into a computer-understandable format.
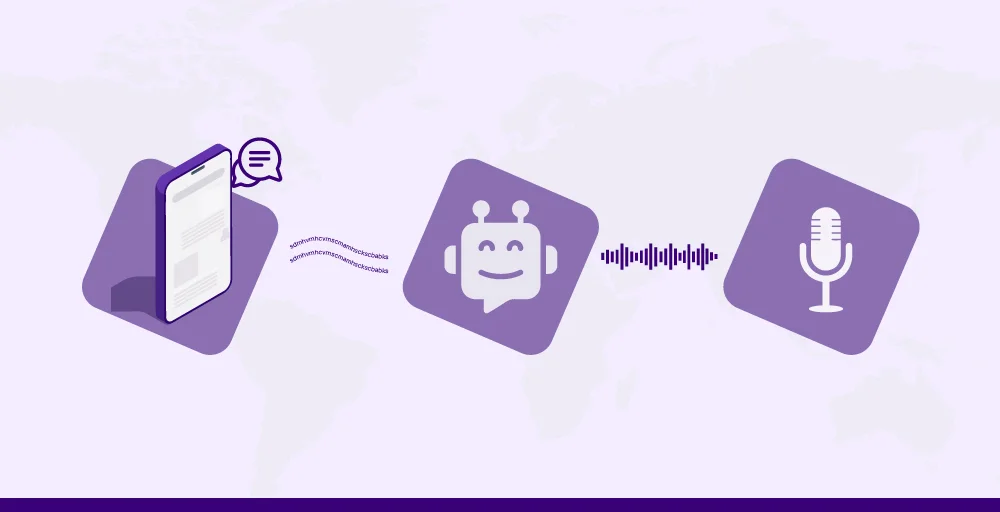
With the help of an Automatic Speech Recognition (ASR) program, people can speak to their computers as they communicate with human beings. The first key takeaway from automatic speech recognition is hearing sound waves and transmitting them into either characters or words.
Automatic speech recognition (ASR) works for any application you might have thought of, from introducing virtual helpers to displaying captions (subtitles) and even background notes while in a hospital, owing to its ability to recognize different accents and dialects. Virtual assistants like Amazon Alexa, Apple Siri, and Google Assistant are facilitated through automatic speech recognition technology.
How does Automatic Speech Recognition Work?
Speech recognition technology translates sounds into written text in a manner that may seem like magic. The four key processes involved are audio analysis, decomposition, digitization, and algorithmic audio matching, with the most appropriate text representation being the most suitable. Thus, spoken words can be changed into computer-readable forms, but text output can still be humanly understandable.
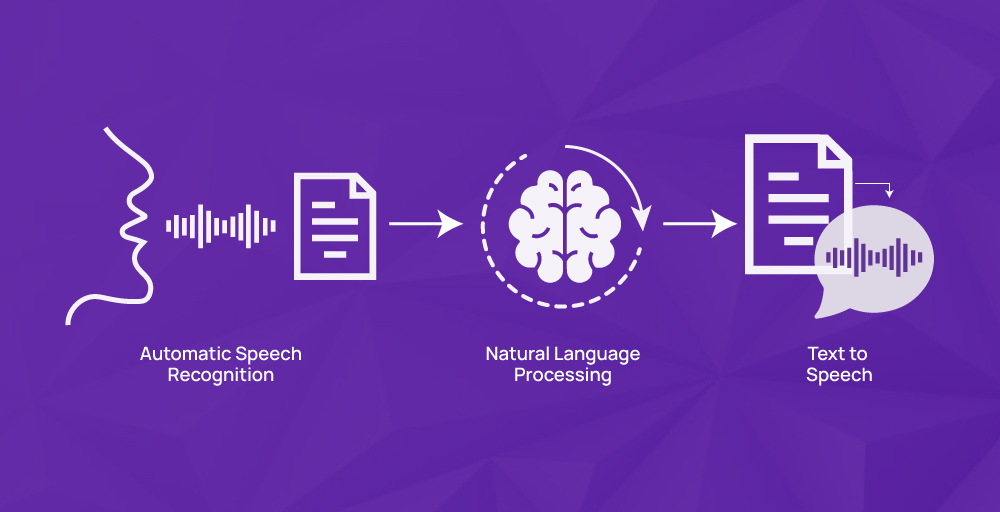
Speech recognition software must adjust to ever-evolving environments to decode human speech. Algorithms used for converting audio recordings into text representations are programmed with different modulations, including dialects, accents, vocal styles, phrasing, and talking patterns, while also tackling issues with any audible sounds surrounding it due to its design.
Automatic Speech Recognition (ASR) voice technologies frequently employ an acoustic model to change audio signals into data that a computer understands. The acoustic model converts your voice into binary information. Language and pronunciation models use computational linguistics to generate words and sentences from each sound in context and sequence.
The traditional hybrid approach
The traditional hybrid ASR approach combines the acoustic model and language model to achieve speech recognition. The first step is to preprocess the audio. The acoustic model decodes and creates an arrangement of possible word sequences, and then the language model rates different word sequences against their linguistic and statistical patterns and confidence scoring to consider the most accurate transcription.
This conventional method has been extensively used in commercial ASR systems because it combines both language and acoustic models, which is an advantage in achieving high recognition accuracy. Even though it has been used extensively, the traditional hybrid approach has drawbacks. Each model must be trained individually, requiring extreme time and labor.
The end-to-end deep learning approach
The end-to-end deep learning approach to speech recognition uses neural networks for directly modeling the incoming audio data rather than using the capturing features from the audio signal and then applying a separate model for recognition as in the traditional hybrid approach. The two main components of most end-to-end deep learning systems for speech recognition are an encoder network that converts raw audio input into a high-level display and a decoder network that generates the final transcription.
This approach is beneficial as it offers end-to-end optimization and is improved at adaptation to new languages or domains. However, including the requirement for vast and varied training datasets and the complexity of the neural network model interpretation can be a challenge.
Benefits of Automatic Speech Recognition Technology
Some of the benefits of ASR technology are:
Increased Productivity
Higher-value tasks get more focus from staff and organizations since transcription of meetings and data entry using voice command or voice dictation tend to save time or energy in addition to other tasks. This is achieved through faster and more accurate conversion of spoken language into text, thus reducing the time spent on activities like voice dictation.
Accessibility for People with Disabilities
ASR technology allows people with disabilities to communicate with computers and phones using their voices. It is designed to help people ask questions or give instructions without using their hands. This will be of great assistance to people whose speech is not clear, those with manual dexterity problems, and those who have difficulty seeing the display.
Improved User Experience
ASR technology improves the use of people’s digital devices. Let us say you have a phone or laptop that supports using ASR technology. Instead of typing or touching it, you can speak on the phone. You can communicate with it by giving commands like “purchase one monitor.” Ask questions like “What is the capital city of Japan?” Furthermore, you can use it to have an oral discussion.
Provide real-time Communication Tools
ASR applications assist in real-time conversations, where individuals can speak and see what is being typed on the other side. This can come in handy if an individual has language difficulties or doesn’t hear well. Importantly, immediate voice translation into text allows for easy comprehension and participation by all individuals in the talk.
Applications of Automatic Speech Recognition
Automatic speech recognition is transforming the way people interact with computers or machines. It has many applications across various fields. Let’s explore some of its applications.
Voice assistants and smart speakers
Automatic Speech Recognition (ASR) technology is used in devices such as voice assistants and smart speakers. Smart speakers like Alexa, Siri, and Google Home are personal assistants that listen to our voice commands.
Google Home or Amazon Echo have home speakers; the vocal assistants can actually hear us speak.
Dictation and transcription services
ASR can also be used for transcription and dictation purposes, wherein it is a high-tech process of orally converting one’s speech into writing. Imagine having a device or software that reads your words and produces them on the screen as symbols of letters.
This is what ASR does. It eases writing and creates documents, archives meetings, discussions, and interviews through transcriptions.
Accessibility for people with disabilities
ASR technology allows individuals with disabilities to access digital devices better. Specifically, it makes the operation of digital devices easier for those who are unable to speak, hear, or see.
Therefore, when a hearing-impaired person says, they can see what another person has told them through transcription in text format. One can also use voice commands to assist those struggling to interact with electronics through their hands.
Call Centers and Customer Service Automation
Call centers are using ASR to automate customer service tasks. On a customer service center’s helpline, you may be asked by an automated voice (IVR) to speak or press some numbers to be connected.
Your speech is interpreted by the ASR, which directs you to the proper division or gives you relevant details. Using this technology, firms can handle many customers’ calls at once and cut down on time wastage.
Advancements in Automatic Speech Recognition
Let’s explore the recent advancements that are shaping the future of automatic speech recognition.
Hybrid acoustic models and multi-task learning
Multitasking while learning and combining different sounds are some advancements in ASR that enable machines to understand and write down spoken words more effectively. Multitask learning and hybrid acoustic models may enhance the vulnerability and competence of ASR systems.
Hybrid acoustic models unite two powerful techniques to make hybrids: relatively closed Markov models and deep neural networks. Apart from the transcription of the voices, ASR also has the capability to understand its speakers or even several languages through multi-task learning.
End-to-end ASR systems and their advantages
ASR system has made translating spoken words into written text simpler. End-to-end ASR systems do not have any pipeline steps thus saving on time for execution and also in model development time.
End-to-end ASR systems, where the system performs all of the operations, are more accurate and simpler. This improves the availability of speech recognition and the efficiency of systems. They use voice assistants or transcription services like Siri on the iPhone, Google Home, Android Auto, etc.
Transfer learning and domain adaptation
Transfer learning utilizes past realization information from models trained to achieve learning processes in newer responsibilities or sectors. Domain adaptation enables ASR systems to be applied to specific domains or based on a specified condition.
Transfer learning and domain adaptation have improved the capabilities and performance of ASR systems in areas like voice dictation or automated answering services like Siri and Alexa.
Attention-based models and their impact on ASR
Attention-based models are an aspect of automatic speech recognition systems that improve accuracy and efficiency. Attention-based models focus on utilizing the characteristics to reproduce a conversation with people’s attention on the specific aspects of the topic.
Attention models have improved ASR by enhancing its ability to allow us to talk to voice assistants, converse, and use speech recognition in our lives.
The Future of Automatic Speech Recognition
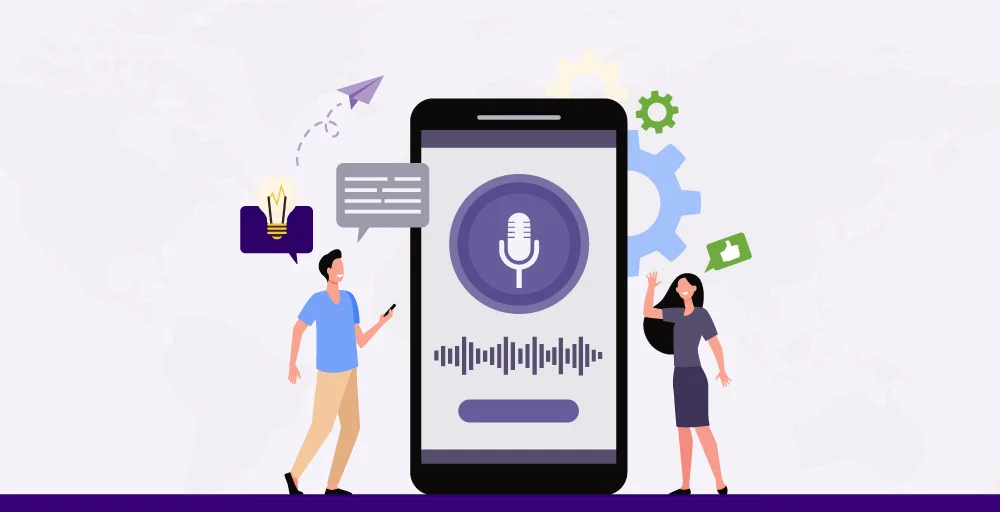
Automatic speech recognition (ASR) is a rapidly developing discipline, and some exciting improvements are planned for the near future.
Enhanced Accuracy
Automated speech recognition’s (ASR) continued growth might involve enhancing its precision. ASR accuracy can be greatly improved by merging with other technologies, such as machine learning and natural language processing. Consequently, ASR could better serve as an accurate tool for interaction between humans and machines in various environments.
More Natural Machine-Human Interactions
Automated speech recognition (ASR) in the future is likely to shift to making human-machine interactions feel more natural. The system’s understanding and responsiveness to verbal communication should be focused on enhancing the natural interaction rather than speech-to-text conversion only. Virtual assistant interactions may seem more lifelike, as though you’re talking with your friend.
Integration with Other Technologies
Integrating ASR with other technologies, such as computer vision, machine learning, AI transcription tools, and natural language processing, will give ASR more power in the future. This mixture of technologies will make the interaction between humans and technology smoother and more natural by improving ASR’s accuracy, flexibility, and adaptability.
Global Accessibility
Making automatic speech recognition (ASR) technology more globally accessible can be crucial to its future development. It is likely that ASR will help many more people other than merely the speakers of an individual language or speakers with certain styles by merging together such things as language translation and multilingual support.
If you are using AI to write content check out above link for ref answer
Challenges and Limitations of ASR
Although automatic speech recognition has significant benefits, it also has challenges and limitations that must be eliminated. Some of the challenges and limitations of ASR are:
- Dealing with background noise and acoustic environments: Background noise can affect speech recognition accuracy, complicating the system’s task of understanding spoken words and successfully transposing them. Various acoustic situations, such as crowded places or rooms with too much echo, distort the speech stream.
- Handling accents, dialects, and speaking styles: ASR systems face challenges and limitations when dealing with accents, dialects, and speaking styles. Accents and dialects exhibited by different people pose problems to ASR models trained on them directly. The authors added that errors in transcribing speeches also occur due to changes in a person’s way of speaking.
- Out-of-vocabulary words and named entities: Automatic Speech Recognition systems may encounter difficulties recognizing named entities and out-of-vocabulary words. It can fail to identify named entities, such as people’s names or specific jargon words. These problems happen because ASR models are often learned on large input sets where all types of vast words and entities are not usually represented.
- Improving accuracy for real-world applications: A significant challenge and limitation of automatic speech recognition systems is to make them more precise for real-world applications. It is difficult to attain high accuracy under many practical conditions, including noise from surroundings, spoken accents, speech pattern changes, and topical languages.
Final Words
The primary concern of Automatic Speech Recognition (ASR) technology is training computers to recognize speech despite its complexity and difficulties. It’s revolutionizing how we already use computers and will continue to do so in the future.
Over the next few years, there may be a self-evident increase in the accuracy and usability of the automated speech recognition system due to the development of new methodologies and technologies. Thus, this will culminate in improved human-to-machine interactions through more natural means and machines with better speech understanding capabilities.
Experience the future of customer engagement with KrispCall!! Don’t miss out; schedule your demo now!
What are some popular ASR tools and applications available?
Some popular ASR tools and applications available are Google Cloud Speech-to-Text, Amazon Transcribe, Microsoft Azure Speech to Text, IBM Watson Speech to Text, Mozilla DeepSpeech, Kaldi, Nuance Dragon, etc.
What is the difference between ASR and speech-to-text?
The difference between ASR and speech-to-text is that ASR focuses on converting spoken language into text. Meanwhile, speech-to-text only focuses on transcribing spoken words into written text.
Which ASR software is best for dictation?
ASR software best for dictation is Nuance Dragon. It is regarded as the best ASR software for dictation due to its high accuracy, advanced customization options, and strong support for various professional use cases, such as medical and legal dictation.